· Dr. Andreas Koeberl · 4 min read
Will AI Co-Workers Make Traditional SaaS Applications Obsolete?
Discover how AI co-workers could transform business operations, potentially making traditional SaaS applications redundant with dynamic workflows, real-time sync, and adaptability.
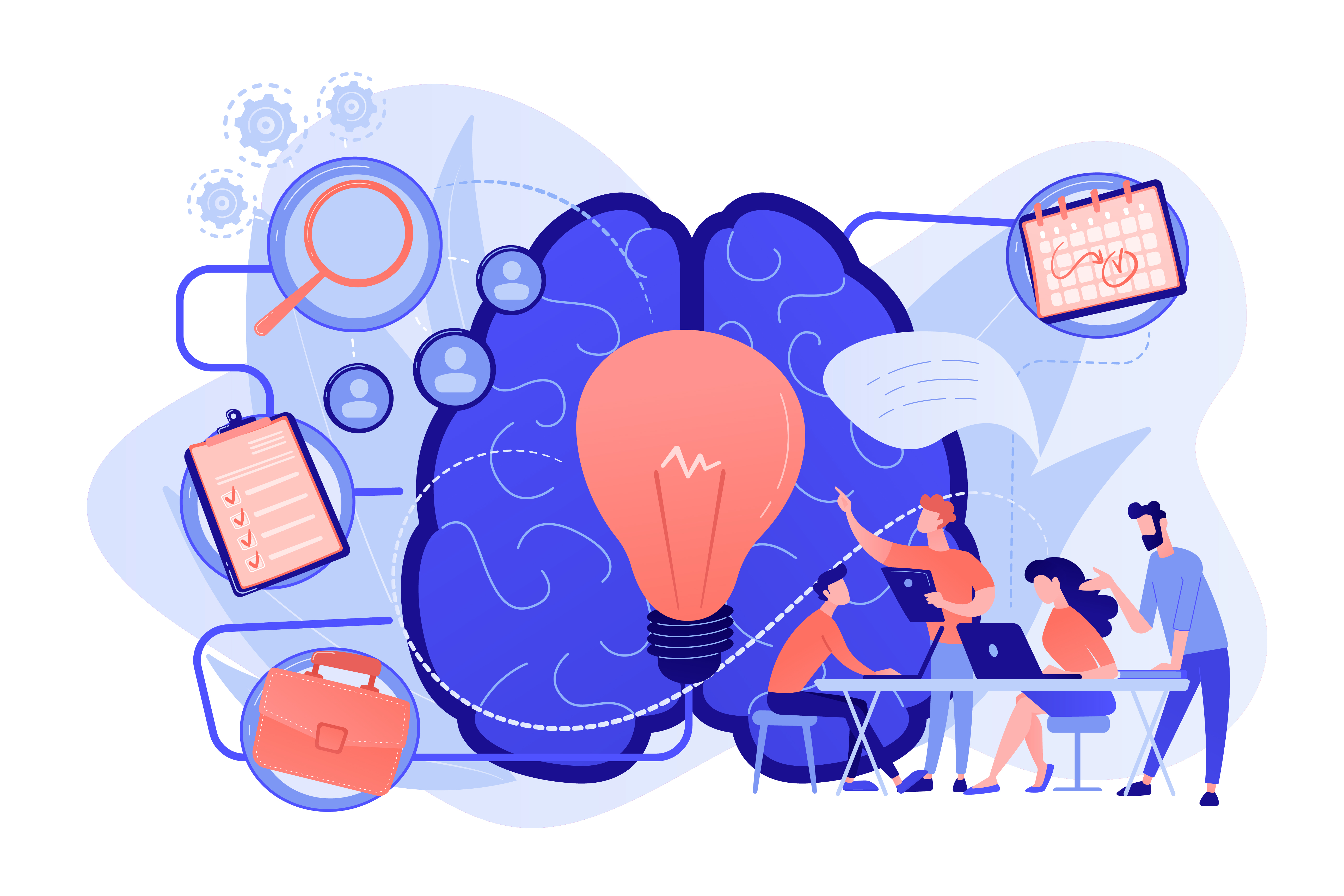
The rapid rise of AI co-workers is reshaping the way organizations operate, leading many to wonder: could these AI employees eventually make traditional SaaS applications obsolete? As technology advances, AI co-workers like Milo from Autonomous Minds are positioned to do more than just assist; they’re evolving into powerful nodes that connect, synchronize, and optimize business processes in ways conventional SaaS applications can’t.
In this post, we’ll explore how AI co-workers integrate with existing tools, dynamically learn workflows, and potentially reduce the need for traditional SaaS applications, especially in smaller, more agile companies.
Connecting the Dots: AI Co-Workers as Central Data Hubs
Unlike traditional SaaS applications that often focus on a single function, AI co-workers are designed to connect seamlessly across various tools and data sources. With access to both (near) real-time first-party data and unstructured static data stored in a vector database (such as chunks of PDFs or documents), these AI agents go beyond typical data integration. Through a central data orchestration layer, AI co-workers can build relationships between disparate systems, “connecting the dots” across all tools and data sources within an organization.
This ability allows AI co-workers to leverage insights from across the company, making data-driven decisions and sharing relevant information across connected systems. It’s a level of connectivity and centralization that traditional SaaS platforms, often operating in silos, can struggle to achieve.
Bi-Directional Sync: Keeping Data Up-to-Date Across All Systems
Elaborating on the inter-connectivity mentioned, one of the resulting critical advantages of AI co-workers is their bi-directional data synchronization. This means that any update made in one data source can automatically trigger updates in all other related tools, with minimal or no human intervention. Imagine a scenario where customer data is updated in the CRM; an AI co-worker can immediately reflect this change across all associated platforms, ensuring data consistency without requiring manual updates.
With fully automated sync capabilities, AI co-workers act as powerful data managers who keep every connected tool and data source up-to-date. This real-time or near-real-time data synchronization reduces errors and ensures that all systems reflect the latest information, removing the common friction points between traditional SaaS systems. This is an essential pre-requisite to ensure the effectiveness of any agentic AI application and a significant advantage of AI co-workers over co-pilots.
Dynamic Workflow Creation: Learning and Improving with Each Goal
Traditional SaaS applications are generally limited to predefined workflows and rules, often requiring manual updates as organizational needs evolve. AI co-workers, however, create dynamic workflows that adapt over time. As these AI agents execute workflows, they learn from each goal (and underlying tasks), gaining insights into how the organization operates. This learning process enables them to suggest and implement continuous improvements for future workflows.
In an ideal setup where data pre-processing is robust, AI co-workers can even adjust workflows automatically based on changes within the organization, such as structural shifts, new products, or leadership transitions. This ability to adapt and learn positions AI co-workers as proactive agents, capable of identifying and suggesting improvements, making them far more versatile than rigid SaaS applications. A simple yet practical example could be new employees joining an organization. An AI co-worker connected to the HR information system would immediately receive the update and adjust workflows (such as approval and information chains) instantly.
Model-Agnostic and Tool-Independent: The Future of Adaptive AI Co-Workers
One of the most groundbreaking aspects of AI co-workers like Milo is that they’re model-agnostic and equipped with the aforementioned versatile data processing layer. This means they aren’t tied to any specific AI model or tool. By building complex relationships across data sources, Milo can function independently of particular tools, effectively adapting to new data sources or processes as they’re introduced. By leveraging universal data models, this could mean that swapping e.g. one CRM tool for another becomes a simple exercise.
This flexibility is a game-changer, as it allows AI co-workers to integrate seamlessly with new systems, reducing dependence on specific SaaS platforms. This adaptability means that companies, particularly small and medium-sized enterprises (SMEs), could streamline their operations by relying on AI co-workers without needing to adopt and manage multiple SaaS applications.
Are Traditional SaaS Applications Becoming Redundant?
Given the capabilities of AI co-workers, it’s possible that certain SaaS applications, especially within SMEs, may become redundant. AI co-workers can handle many of the functions traditionally managed by SaaS applications—like CRM, project management, or data analysis—while offering deeper insights, real-time synchronization, and adaptability that traditional SaaS can’t provide easily.
While there are limitations in larger organizations with e.g. complex intercompany connections or strict regulatory requirements, as AI co-workers like Milo continue to evolve, their role in business operations will likely expand, offering hyper-connected, adaptive solutions that reduce the need for multiple standalone applications. For agile SMEs, this shift could mean a significant reduction in software complexity and costs, leading to a more streamlined and efficient work environment.